Project overview
Our client, a steel plant, was facing recurrent performance issues on a shear, resulting in significant asset downtime.Shears are used in steel-making process to cut the steel bars to the desired length. Understandably, downtime on this critical equipment can have drastic impacts on productivity as it creates a bottleneck in the process.
With 110 hours of unplanned downtime per year, 20% of which was caused by mechanical or electrical issues, reducing shear downtime became a priority to keep the plant profitable.
If our client could reduce the shear’s downtime by half, the entire plant’s downtime would be reduced by 10%.
Unfortunately, the maintenance programs initially in place were not effective in preventing failures. To help our client achieve their goals, the team began the process of redesigning and optimizing preventive and predictive maintenance. This PM consolidation process was the starting point for the implementation of the innovative solution.
A shift in approach was in order.
Instead of repairing an asset when it breaks or scheduling maintenance at regular intervals – both methods implying unplanned downtime – a predictive approach involves monitoring an asset’s health at all times in order to predict stay ahead of the curve.We went one step further and developed a smart tool based on machine learning and prescriptive analytics, to predict failures before they happen and prescribe actions to prevent them.
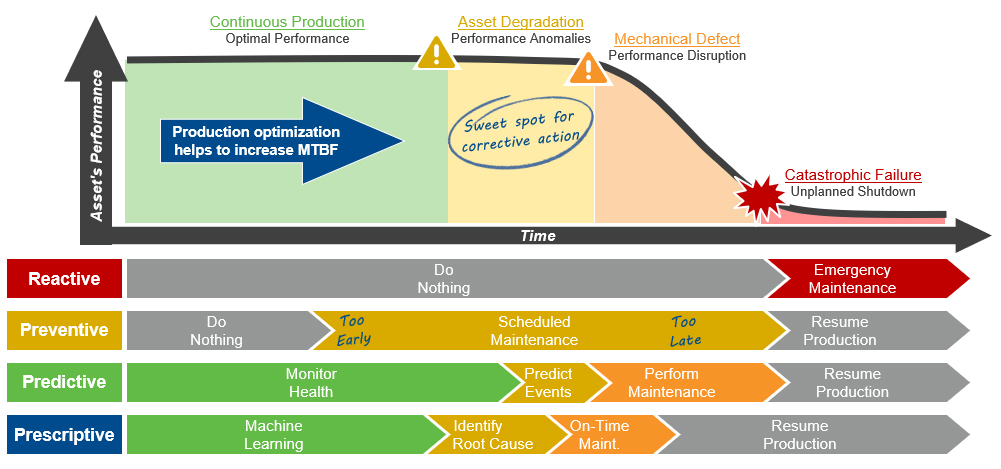
An algorithm to the rescue
Our experts used machine learning to extract, clean, transform and analyze operational and manufacturing data from the asset.Using libraries of advanced statistical and machine-learning algorithms, the solution consumes large quantities of diverse information technology (IT) and operational technology (OT) data into actionable knowledge that drives real-time decisions to improve process and asset performance.
Characteristics :
- Expert rules combining calculations, logic, temporal reasoning, statistical and first principal models to detect and predict complex events and abnormal conditions
- Abnormal conditions management based on fault models and root cause analysis
- Predictive models deployed online to estimate key indicators and to recognize patterns and abnormal conditions
- Actionable knowledge online in forms of pertinent corrective actions
- Integration platform to collect real time and historical process data and events from the plant’s databases
With the solution installed, commissioned, and operating, the results are currently being monitored. Downtime was reduced by 50 hours in 2020 and maintained in 2021, generating a significative positive impact on the bottom line: we estimate the savings for the first 3 years of use to be $1M.
Transform your industrial challenges into results!
Fill the form below to start the conversation.